As one of the final blog posts of the semester, I felt that it would be prudent to do one last overview of some developing technologies. There are 5 different emergent technologies that I believe are likely to become increasing influential in the coming years: CRISPR gene editing technology, XR hardware and software, Artificial Intelligence, Cloud Computing, Specialized Medicine.
CRISPR
Emmanuelle Chapentier (Left) and Jennifer A. Doudna (Right) are the 2020 recipients of the Nobel Prize in Chemistry for their work on CRISPR gene editing technology
Emmanuelle Charpentier and Jennifer A. Doudna were the recipients of the Nobel prize for chemistry in 2020, and already the technology has begun to revolutionize science and medicine. The premise of CRISPR is that it takes advantage of a pre-existing pathway in bacteria that evolved as an immune response defense against viruses and bacteriophages. In essence, it keeps a record of the viral DNA it comes across in the past. When it runs into that same DNA again, it synthesizes a protein complex that seeks out and breaks the viral DNA in half — thereby ensuring its destruction and protecting the cell. Now, the applications of CRISPR are unlikely to be readily apparent from that description alone; the real magic happens in that it enables scientists to purposefully break DNA sequences in half and insert DNA that they want to be there instead: genetically modifying the host cell.
This kind of targeted gene editing wasn’t possible before CRISPR, and it will only get better as time goes on. While there are many positive use cases for this technology — gene therapeutics for genetic diseases — it can just as easily be used to carry out oppressive practices in society (watch the movie Gattaca if you’re curious).
Related to this kind of low-level modifications to the genome is the emergence of increasingly specialized medicine and healthcare.
Specialized Medicine
The human genome has been fully sequenced and annotated. Every day we get closer to understanding how each part of it contributes to the creation of each variation of expression. DNA Sequencing has become cheaper and more feasible. The genome of an entire person can be sequenced in about a day.
Once again, DNA sequencing technology is a dual use tool. While it can be used for more specialized healthcare, improving the quality of life of many, it could also be used as a determinant in one’s freedom and mobility in society. Once again, I’ll call upon the example of Gattaca.
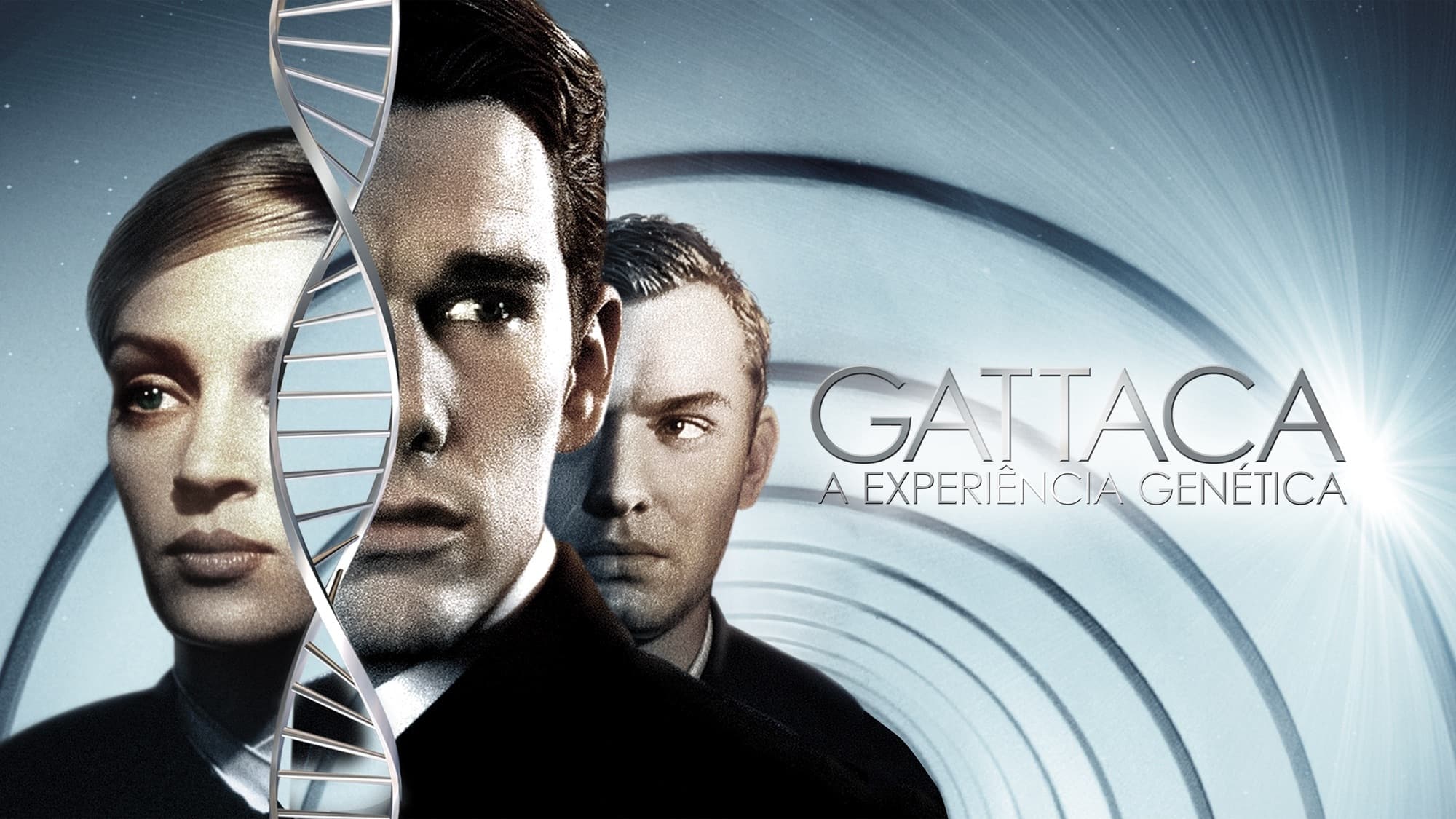
Poster for the film Gattaca. A film about a dystopian future in which your genome determines your entire life.
In the film, people are able to determine, with near 98% certainty when someone is likely to die, solely based on the content of their genome. As this information is freely accessible by anyone who can obtain a DNA sample, employers use it as a key determinant in the hiring of an applicant — are they healthy enough? How expensive will insurance be? Are they worth the investment?
While it may be exaggerated in the film, these are becoming increasingly pressing issues when one takes into account the rise of machine learning technologies, tools specialized in the interpretation of large amounts of data. What are you going to do when a ML model says that you aren’t fit to live somewhere based solely on the content of your bloodstream and a 98% accuracy?
Artificial Intelligence and Machine Learning
As I’ve discussed a multitude of times throughout this blog series, machine learning and artificial intelligence are very powerful emergent technologies that will be key determinants in our future. Such powerful tools should not be left unchecked and in the hands of the few. I believe that regulation is necessary — immediately — before large “Big Data” companies turn us into their product, harvesting our information, feeding it into an algorithm, and selling the product back to us as if it’s something we didn’t own in the first place.
XR
Speaking of “Big Data” companies, let’s delve into the Metaverse for a second. While grossly overhyped when Meta initially announced their name change, I believe that XR (a term that covers Virtual Reality and Augmented Reality) has its place amongst the technologies that will shape our future. Currently, most of the available devices and screens are confined to two dimensions, we interact with flat screens as a way of communicating — imagine how dramatic a change to three dimensions will be?
While there are many dystopian aspects to the Metaverse that Meta is trying to sell to people, I believe that the creative aspects of Virtual and Augmented Reality are not to be undersold. The emergence of two dimensional images led to the eventual rise of 2D movies and the film industry.
Think about how much the film industry has shaped entertainment over the past century, now imagine how crazy it’ll get when studios are able to produce three dimensional movies and stories. Perhaps instead of being a simple observer, you get to shape the story yourself — who knows.
The two dimensional film industry is so well established that there is a clear methodology for how to create a movie, it’s so engrained in the culture that it seems obvious now. However, few people realize how experimental the early years of film production were as people struggled to figure out what worked — I believe that we are going to see something very similar with VR and AR in the coming decade.
Cloud Computing
Save your data in the cloud is a phrase that we’ve all heard before. It’s more protected, as shown by how Ukrainian cybersecurity is bolstered by cloud based servers, and it’s more powerful as you are able to take advantage of more advanced machinery. Looking at the revenue Amazon has generated through server hosting highlights our growing dependence on cloud based technologies. This will only get more amplified in the future as more people flock to AI development and fight for the scarce GPU resources provided by retailers.
These are just some of the fields that I find interesting. I believe that will be increasingly important in the future, but then again, some of the most revolutionary technologies have been those that people weren’t even aware of a year before — creating an entirely new field for innovation.