Scalable Artificial Intelligence for Aerospace Design
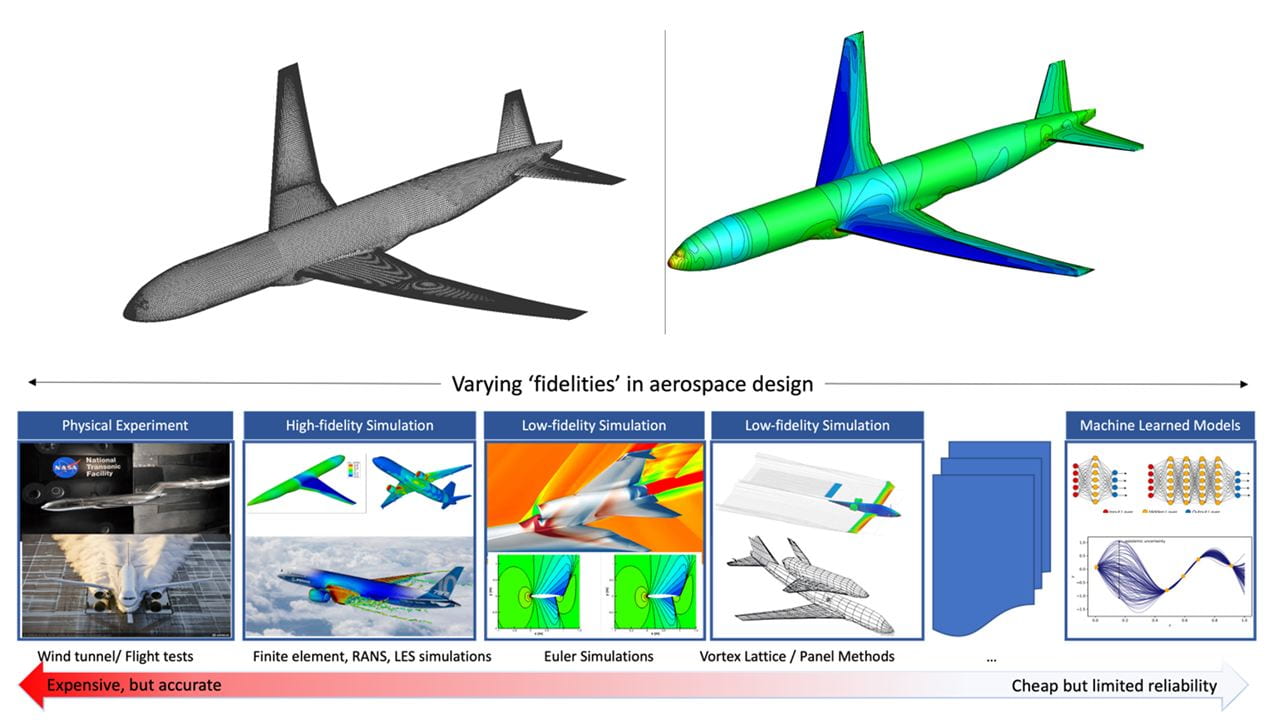
UNIVERSITY PARK, Pa. – The design of aerospace systems, from entire aircraft to sub-systems such as aircraft engines, is time consuming and expensive. For example, the development of the Boeing 787 Dreamliner cost $22 billion and needed almost a decade. The root cause of such staggering costs is that the existing paradigm of aerospace design depends on a cycle of design decisions driven by intuition alternating with extensive analysis. This process tends towards only incremental improvements to current designs. The Computational Systems Design Laboratory (CSDL), led by Ashwin Renganathan, strives to make aerospace decision-making highly data-driven by leveraging high-fidelity physics-based simulations, high-performance computing (HPC), and artificial intelligence (AI).
The existing paradigm in aircraft design is carried out in three stages: conceptual, preliminary, and detailed design. The conceptual stage is where major design decisions are made, such as number of engines, shape of the fuselage, and placement of the wings and tail. However, the performance and safety of the design are rigorously assessed only during detailed design; any major concerns revealed in this later analysis require re-doing significant parts of the earlier stages. CSDL seeks to enable data-driven, rigorous decisions early in design through two key developments. First, high-fidelity models (e.g., computational fluid dynamics), enabled by high performance computing, offer realistic evaluations of aircraft performance earlier in the design process at a fraction of the cost of physical prototyping. Second, AI can “learn” to predict what high-fidelity models would calculate at a fraction of the computational cost.
The CSDL at Penn State leverages high performance computing (supported in part by Penn State’s Institute for Computing and Data Science), high-fidelity models of vehicle performance, and AI to develop scalable design tools supporting economical, fast, and yet reliable decisions in the presence of uncertainty. In one of the ongoing projects at CSDL, Renganathan and his students use AI to develop “multifidelity” methods, where decision-making is driven by multiple disparate sources of information. For instance, high-fidelity computational aerodynamic simulations of aircraft can still be prohibitively expensive to test beyond a few dozen design alternatives, even as they provide the most realistic estimate of aircraft performance. Cheaper, “lower fidelity” may not be completely accurate but can still provide approximate estimates of performance. Using probabilistic multifidelity methods driven by AI, Renganathan’s group developed automated tools that significantly reduces the cost of decision-making by exploiting cheaper low-fidelity models for less critical design decisions while sparingly querying the expensive high-fidelity models.
These tools and AI models developed at the CSDL are founded on three areas in applied mathematics: approximation theory, uncertainty quantification, and optimization. Renganathan’s appointment as an Institute of Computational and Data Sciences co-hire uniquely supports him with computational resources that enable both high-fidelity physics-based aircraft simulations as well as training and deploying large-scale AI models.
– November 30, 2023 -ARP and AR