Trying very hard to produce the “desirable” results through data analysis can be a great danger. The federal Office of Research Integrity defines three types of research misconduct: fabrication, falsification, and plagiarism (Office of Research Integrity, 2017). In particular, falsification refers to “manipulating research materials, equipment, or processes, or changing or omitting data or results such that the research is not accurately represented in the research record.” According to this definition, falsification can happen prior to, during, or after data collection. In practice, falsification often takes place after data collection; that is, much of falsification happens during data processing, statistical analysis, and representation of the results.
2.1 Data fishing and trimming
A fisherman would not mind using multiple hooks to catch a big fish, but the same strategy might be problematic in data analysis. DeMets (1999) uses “data fishing” to describe the “conducting [of] endless analyses until one statistically significant result is found.” Because a size-able dataset might contain random correlations, repetitive analysis of the same set of data might accidentally reveal “statistically significant” correlations between certain variables. Because these seemingly correlated relations come into being purely by chance, they should not be reported as research findings.
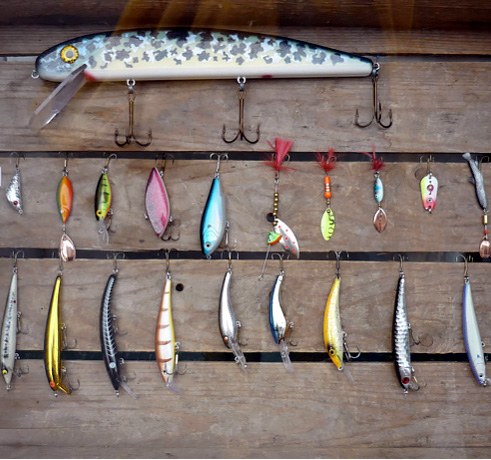
Figure 3 Multi-hooks for Fishing.
(Rapala lures by Fanny Schertzer / CC BY-SA-3.0)
Data trimming is another problematic practice in analyzing research data. Data trimming happens when the researcher selectively abandons part of the data that is considered invalid because it is not collected following the protocol. An example of data trimming would be removing data contributed by unqualified participants after data collection is complete. DeMets (1999) points out that data trimming might introduce substantial biases in the statistical analysis, for such actions compromise the randomness of the data set.
One may not consider data fishing or data trimming as falsification, because neither practice explicitly changes the value of the raw data. Indeed, in rare cases, these practices can produce valid results. For example, repetitive analysis of large data sets might detect correlations we did not anticipate in advance. However, deliberately fishing or trimming data in order to produce statistically significant results is an act of misrepresenting the research, and it should be avoided.
2.2 Image manipulation
With the increasing use of graphics in research publications, the research community has become alerted to an additional type of misconduct: image manipulation. In 2005 and 2006, 44% of research misconduct cases opened by the Office of Research Integrity involved image manipulation (Parrish and Noonan, 2009). Many researchers understand that some degrees of image processing is valid and even necessary for enhancing its readability, but one should be careful that image “beautification” can be overdone. Parrish and Noonan (2009) cite seven forms of image manipulation identified by Rossner and Yamada (2004): “(1) gross misrepresentation, (2) brightness/contrast adjustments, (3) cleaning up the background, (4) splicing lanes together, (5) enhancing a specific feature, (6) linear versus non-linear adjustments, and (7) misrepresentation of a microscope field.” The boundary between appropriate and inappropriate image processing can be blurry, and it often depends on the discipline, methods, and objects of study. According to Parrish and Noonan (2009), misconduct cases may involve “(1) image enhancement, accentuating or diminishing attributes of the taken image; (2) presenting an image as something other than what it is; and (3) altering an image.”
Next Page: Previous Page: